Introduction
Predictive analytics offers several benefits in property markets. It enables developers, investors, and buyers to assess potential risks and opportunities.
By analyzing historical data and current market conditions, predictive analytics can identify trends and predict fluctuations in property prices.
This information allows stakeholders to make strategic decisions regarding property investment and development.
One of the key benefits of predictive analytics in property markets is its ability to forecast demand and supply dynamics.
This information is crucial for developers who need to determine the optimal size, pricing, and location of new projects.
By analyzing variables such as population growth, income levels, and consumer preferences, predictive analytics can estimate future demand and guide developers in meeting market needs.
Additionally, predictive analytics can help property investors identify investment opportunities and maximize returns.
By analyzing factors such as rental yields, occupancy rates, and macroeconomic indicators, investors can make informed choices about which properties to invest in and when to buy or sell.
Lastly, predictive analytics can assist buyers in making informed decisions about purchasing or renting properties.
By analyzing factors such as neighborhood characteristics, property features, and market trends, buyers can evaluate the long-term prospects and value of potential investments.
In essence, predictive analytics plays a vital role in property markets by providing valuable insights and predictions.
Its ability to forecast future trends and outcomes empowers stakeholders to make informed decisions, minimize risks, and optimize returns.
As technology continues to advance, the use of predictive analytics in property markets is expected to become increasingly prevalent and influential.
Applications of Predictive Analytics in Property Markets
Forecasting property prices and market trends
Predictive analytics plays a crucial role in the property market through various applications.
By utilizing historical data and statistical models, property prices and market trends can be forecasted accurately.
Transform Your Real Estate Decisions
Unlock personalized real estate insights crafted just for you. Get actionable advice designed to amplify your success.
Get StartedThe identification of patterns and factors influencing property prices helps professionals project future movements, assisting investors and buyers in making informed decisions.
Predicting demand and market saturation
Predictive analytics is also highly valuable in predicting demand and market saturation.
By analyzing population growth and economic indicators, professionals can predict the demand for properties in specific areas.
This analysis enables them to identify regions with high demand potential, allowing investors to focus their resources accordingly.
Additionally, assessing the risk of oversupply helps prevent investment in saturated markets, avoiding potential losses.
Assessing investment and development opportunities
The technology further assists in assessing investment and development opportunities in property markets.
Professionals can evaluate potential returns on investment by analyzing market trends and historical data.
Data-driven insights help identify emerging markets and profitable locations, increasing investment success rates.
Moreover, assessing market risk and investment feasibility enables individuals to make well-informed decisions about various property ventures.
In fact, predictive analytics offers significant applications in property markets.
Accurate forecasting of property prices and market trends allows investors and buyers to make informed decisions.
Predicting demand and market saturation helps investors focus resources on high-demand areas and avoid oversaturated markets.
Assessing investment and development opportunities mitigates risk and ensures profitable ventures in the ever-evolving property market.
Read: AI’s Role in Streamlining Real Estate Sales
Showcase Your Real Estate Business
Publish your company profile on our blog for just $200. Gain instant exposure and connect with a dedicated audience of real estate professionals and enthusiasts.
Publish Your ProfileTechniques and methodologies used in predictive analytics in property markets
Predictive analytics in property markets involves the utilization of various techniques and methodologies to predict market trends, prices, and demand accurately.
These techniques and methodologies are crucial in providing valuable insights to stakeholders.
Data collection and cleansing
The first technique used in predictive analytics is data collection and cleansing.
This involves gathering relevant property data and market indicators that have a significant impact on property prices.
The data collected can include factors such as location, size, amenities, and economic indicators.
Once gathered, the data is cleaned and preprocessed to eliminate any inconsistencies, errors, or missing values that could affect the accuracy of the analysis.
Statistical modeling and machine learning
Statistical modeling and machine learning are essential in predictive analytics.
Regression analysis is commonly used to forecast property prices based on historical data and relevant market indicators.
By identifying the relationship between independent variables and the property’s price, regression analysis can generate predictive models that help anticipate future price movements.
Time series analysis is another technique used to identify trends in property markets.
By analyzing historical market data over time, patterns and patterns can be identified, allowing stakeholders to make informed decisions.
Time series analysis helps predict future trends, enabling investors to make strategic moves in the market.
Machine learning algorithms play a crucial role in demand prediction.
By analyzing historical demand data and considering various factors such as economic conditions, population growth, and market dynamics, machine learning algorithms can predict future demand for specific properties.
This information is valuable to property developers, investors, and other stakeholders in the property market.
Data visualization and interpretation
Data visualization is an integral part of predictive analytics, as it allows stakeholders to understand complex data easily.
Creating visual representations, such as charts, graphs, and heatmaps, helps in identifying patterns, relationships, and outliers in the analyzed data.
These visual representations make it easier to interpret data and gain insights into market trends and patterns.
Interpreting the results and insights gained from predictive analytics is crucial for making informed business decisions.
By understanding the implications of the data analysis, stakeholders can identify potential investment opportunities, predict market fluctuations, and optimize their strategies.
Effective interpretation of results leads to more successful real estate investments and increased profitability.
Communicating findings effectively to stakeholders is essential in predictive analytics.
Stakeholders need to understand the insights and recommendations derived from the analysis to make informed decisions.
Presenting the findings in a clear, concise, and visually appealing manner ensures that the information is easily digestible and actionable.
In short, predictive analytics in property markets utilize various techniques and methodologies to provide accurate predictions and valuable insights.
From data collection and cleansing to statistical modeling, machine learning, and data visualization, each step is crucial in understanding market trends, forecasting prices, and predicting demand.
The effective utilization of these techniques empowers stakeholders to make informed investment decisions, optimize strategies, and maximize profitability in the property market.
Read: Navigating Real Estate with AI Assistance
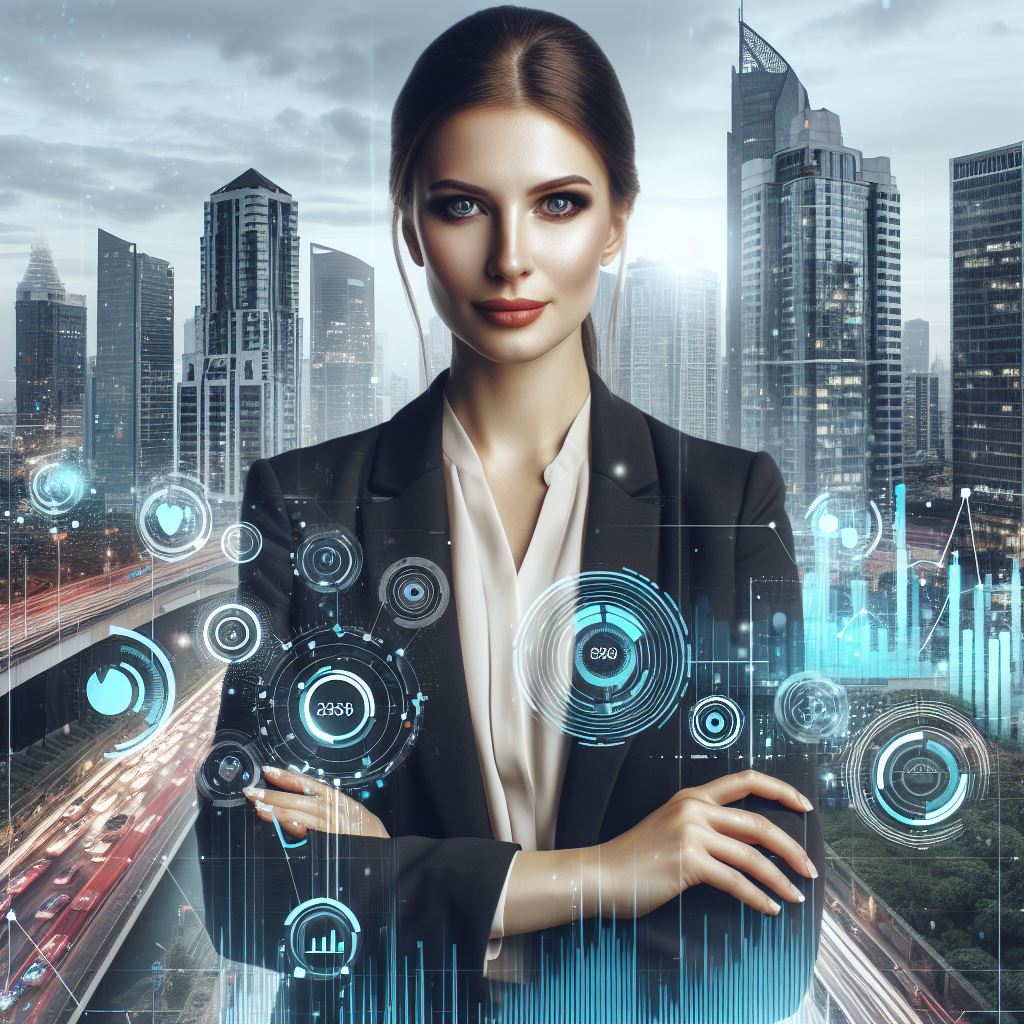
Challenges and Limitations of Predictive Analytics in Property Markets
Predictive analytics has emerged as a powerful tool in analyzing property markets and making informed decisions.
However, it is important to acknowledge the challenges and limitations associated with this approach.
Showcase Your Real Estate Business
Publish your company profile on our blog for just $200. Gain instant exposure and connect with a dedicated audience of real estate professionals and enthusiasts.
Publish Your ProfileData availability and quality
One significant challenge is the limited availability of comprehensive property data.
Obtaining detailed information on properties, including their characteristics, prices, and historical data, can be a complex and time-consuming process.
Additionally, there may be variations in data quality, making it difficult to rely on the accuracy and reliability of the available information.
Uncertainty and external factors
Another challenge arises from the influence of unforeseen events on market dynamics.
Predictive analytics models heavily rely on historical patterns and trends, but unexpected events such as natural disasters or economic crises can significantly disrupt the property market.
These external factors introduce uncertainty and can render predictive models less reliable.
Economic, political, and environmental factors also have a substantial impact on property markets.
Changes in government policies, fluctuations in interest rates, or shifts in environmental regulations can profoundly affect property values and market dynamics.
Predictive analytics models may struggle to incorporate these complex external factors and accurately forecast market trends.
Ethical considerations and biases
While predictive analytics can provide valuable insights, it is crucial to address ethical considerations and biases in the development and deployment of these models.
Fairness and avoiding biases are essential to ensure that predictions do not discriminate against certain groups or perpetuate existing inequalities.
It is challenging to eliminate biases entirely from predictive models, as they are often trained on historical data that may reflect historical prejudices or disparities.
Striving for fairness requires careful consideration and evaluation of the features and variables used in the predictive models to identify and address potential biases.
Furthermore, balancing privacy concerns with data utilization is another ethical consideration.
Predictive analytics requires extensive data collection and analysis, raising concerns about privacy violations.
It is essential to handle personal information responsibly and ensure compliance with privacy regulations.
Overall, predictive analytics in property markets faces challenges related to data availability and quality, uncertainty caused by external factors, and ethical considerations.
While these limitations exist, they can be mitigated through continued advancements in data collection, model development, and ethical frameworks.
Predictive analytics still holds great potential to improve decision-making in property markets.
Read: AI: A Game Changer in Home Buying & Selling
Case Studies of Successful Predictive Analytics Implementation in Property Markets
These case studies highlight the successful implementation of predictive analytics in property markets.
By utilizing data from various sources and employing advanced algorithms, cities like Boston and platforms like Zillow have achieved valuable insights and improved decision-making processes.
Example 1: Boston’s use of predictive analytics with CityScore to identify undervalued neighborhoods
Boston’s CityScore initiative demonstrates how the use of predictive analytics can identify undervalued neighborhoods.
By combining data from different city departments, service requests, and social media, Boston obtains a comprehensive view of the city’s performance.
Machine learning algorithms analyze this data to identify patterns and predict future trends.
This information enables property investors to make targeted investments in neighborhoods poised for growth.
Moreover, it allows authorities to prioritize revitalization efforts to areas in need, promoting balanced urban development.
Example 2: Zillow’s use of predictive modeling for property price predictions
Zillow, on the other hand, showcases the use of predictive modeling to forecast property prices.
Through regression models and machine learning algorithms, Zillow considers various factors like location, market trends, and historical sales data.
These factors are weighted and analyzed to generate predictions for individual properties.
Showcase Your Real Estate Business
Publish your company profile on our blog for just $200. Gain instant exposure and connect with a dedicated audience of real estate professionals and enthusiasts.
Publish Your ProfileWhile Zillow’s predictions are generally accurate, they emphasize the influence of external variables, such as economic conditions or unforeseen events, that can impact accuracy.
Continuous updates and user feedback help them refine their models and improve prediction reliability over time.
Overall, these case studies demonstrate the power and potential of predictive analytics in property markets.
By harnessing the vast amount of data available and leveraging advanced algorithms, cities and platforms can make informed decisions, improve urban planning, and provide valuable insights to property investors.
As technology continues to advance, predictive analytics will likely play an even more significant role in shaping property markets and promoting sustainable development.
Read: Tech-Ready Homes: A Must for Modern Buyers
Conclusion
Recap of the importance and benefits of predictive analytics in property markets
Predictive analytics play a crucial role in property markets, providing valuable insights and predictions that can help professionals make informed decisions.
By analyzing historical data, market trends, and other relevant factors, predictive analytics can identify potential risks and opportunities, assist in pricing and forecasting, and optimize resource allocation.
Emphasizing the need for continuous improvement and innovation in data analytics
It is essential for real estate professionals to constantly update and refine their predictive analytics models.
Markets are ever-changing, and new data sources and techniques are constantly emerging.
By staying up-to-date with these advancements, professionals can ensure that their predictive models remain accurate and reliable.
Encouragement for real estate professionals to embrace predictive analytics for strategic decision-making
Predictive analytics can help real estate professionals gain a competitive edge by enabling them to make data-driven decisions.
By leveraging predictive models, professionals can anticipate market trends, optimize investment strategies, and identify potential risks before they occur.
By embracing predictive analytics, real estate professionals can effectively navigate today’s dynamic property markets and achieve long-term success.